- 1Department of Medical Oncology, Szent Lázár County Hospital, Salgótarján, Hungary
- 2Department of Economical and Financial Mathematics, University of Debrecen, Debrecen, Hungary
Introduction: Consistent association between elevated baseline serum values and C-reactive protein (CRP), cross-linked fibrin degradation products (D-dimer), lactate dehydrogenase (LDH), decreased baseline serum albumin, absolute lymphocyte count to absolute monocyte count ratio (LMR), elevated absolute neutrophil count to absolute lymphocyte count ratio (NLR), elevated platelet count to absolute lymphocyte count ratio (PLR), and between some combinations of these biomarkers and the short overall survival of patients with malignant diseases has already been reported. These biomarkers are independent prognostic factors for cancer. Here, the most significant biomarker combination of these values was searched and studied in real-life advanced cancer patients of a single center.
Methods: The authors retrospectively analyzed the association of the aforementioned biomarkers and their combination and OS of 75 consecutive cancer patients with locally advanced, recurrent, or metastatic diseases. Validated cut-off determination was used.
Results: CRP, albumin, and PLR showed marked association with OS. Cut-off values for significant shorter OS were 30.65 mg/L (p < 0.001), 44.35 g/L (p < 0.001), and 168.20 (p < 0.001), respectively. Based on assessed biomarker cut-offs, four patient groups were created to determine whether biomarker values were out of range (ORV) compared to cut-off: 1) No ORV biomarkers (n = 24; OS = 26.07 months); 2) one ORV biomarker (n = 21; OS = 13.50 months); 3) two ORV biomarkers (n = 20; OS = 7.97 months), and 4) three ORV biomarkers (n = 10; OS = 3.91 months). Significant differences in OS were detected between the groups: For 1. vs. 2. hazard ratio (HR) = 3.0 (95% CI: 1.5–6.2), p = 0.003; for 1. vs. 3. HR = 4.1 (95% CI: 2.0–8.3), p < 0.001; and for 1. vs. 4. HR = 10.2 (95% CI: 4.2–24.6), p < 0.001.
Conclusion: Based on our analysis, we can confirm that the complex monitoring of CRP, albumin, and PLR would provide a good estimation of OS. Large scale prospective studies are warranted to explore this and other useful combinations of prognostic biomarkers and their relationship to the well-established prognostic systems in real-life.
Introduction
Some routinely measured laboratory analyte baselines have been shown to have prognostic importance in malignant diseases. Both prospective and retrospective studies and also meta-analyses have described the poor prognostic role of elevated baseline C-reactive protein (CRP) (1–4), cross-linked fibrin degradation products (D-dimer) (5–8), lactate dehydrogenase (LDH) (9–12), and decreased albumin (13–15) in cancer. Deme and Telekes have also reviewed the value of elevated CRP (16), D-dimer (17), LDH (18), and decreased albumin (19) for poor outcomes of cancer patients. Decreased lymphocyte to monocyte ratio (LMR) is a factor for adverse prognosis in several cancers (20–24). Based on a large scale (25) and further smaller meta-analyses (26–33), a high absolute neutrophil count to absolute lymphocyte count ratio (NLR) has also been associated with short overall survival (OS) in many solid malignant diseases. Elevated platelet count to absolute lymphocyte count ratio (PLR) was also shown to be an adverse prognostic factor in various cancers (34–45).
Here, we evaluated the associations of baseline CRP, D-dimer, LDH, albumin, LMR, NLR, and PLR with the outcome of 75 consecutive patients with advanced cancer suitable for anticancer therapy, i.e., Eastern Cooperative Oncology Group (ECOG) performance status ≤ 2. Our hypothesis was that we could find the combination of the most significant biomarkers, which would provide accurate prediction for OS in a real-life setting, and the results may confirm the data of the literature.
Materials and Methods
Patients
Blood samples of consecutive patients with locally advanced, recurrent, or metastatic malignant diseases were taken in our clinical chemistry laboratory (Szent Lázár County Hospital, Salgótarján, Hungary) as part of the routine investigation before the initiation of the therapy of the given disease. Obvious symptoms and signs of common infectious diseases were assessed (purulent cough, pulmonary crackles, or symptomatic bacteriuria). Exclusion criteria included suspected infection, hematological malignancy, the lack of at least one biomarker data point, rapid progression (i.e., from laboratory testing, ECOG performance status progressed to 3 before the initiation of anticancer treatment), or death caused by something other than disease progression. Patients with all the following biomarkers available were included in the study: CRP, D-dimer, LDH, albumin, and complete blood count (CBC). Data of 13 excluded patients are given in Supplementary File S1.
Methods
CRP, LDH, and albumin were measured with commercially available Roche tests on Cobas c501 or Cobas 6000 analyzers (Tokyo, Japan). D-dimer levels were measured by a chemiluminescent immunoassay (PATHFAST, Tokyo, Japan). CBC was determined with Cell-dyn 3700 (Abbott Park, IL, United States and Beckman Unicel DxH600, Miami, FL, United States). The LMR, NLR, and PLR were calculated as the ratio of the lymphocyte count and the monocyte count, the ratio of the neutrophil count and the lymphocyte count, and the ratio of the platelet count and the lymphocyte count, respectively.
Statistical Analysis
For the purpose of statistical analysis, we used for CRP <5 mg/L (lower level of detection), the value of 4.9 mg/L, and for D-dimer >5 mcg/mL (higher level of detection), the value of 5.1 mcg/mL. All other biomarker values were handled with the measured numeric values. Cut-off determination was performed with the validated “Cutoff Finder” online tool (46). After uploading the tab separated value file (Supplementary File S2), for each biomarker the “Survival Time” was OS or censored OS, the “Survival Event” was the variate of 1 for OS or 0 for censored OS, and the “Method for cut-off determination” was “Survival: significance (log-rank test). Statistical analysis was performed by R Studio Software (47). Semicolon separated value file (Supplementary File-2b.csv) was used.” For each value a comparison was made between the median OS values below and over the cut-off value by the log-rank test. The value with the largest gap and Chi-squared statistics was selected. Comparison of the prognostic groups with Cox proportional hazard regression was performed. Log-rank test was used to detect the differences between survival curves within the prognostic groups in the Kaplan-Meier analysis as well as to assess the significance of the Cox model. Effect size estimation was performed for the Mann-Whitney probe by calculating the so called Eta-squared value. Between 0.06 and 0.14, the effect can be considered medium-sized, while over 0.14 it can be considered large. Power analysis was performed with the “powerCT” function in the “powerSurvEpi” package of the R Studio software. All figures were drawn as vector graphics in Scalable Vector Graphic format in the “ggsurvplot” and “ggforest” functions in the “survminer” package of the R Studio software (47) and edited by Inkscape software (https://inkscape.org). The R-script is available in Supplementary File S3.
OS time was defined as the length of survival from the date of laboratory testing. Survival data measured in months were computed according to Surveillance, Epidemiology, and End Results (SEER) recommendations (https://seer.cancer.gov/survivaltime/SurvivalTimeCalculation.pdf): days between the dates were divided by one twelfth of 365.24. For the median follow-up time calculation, we used a reverse Kaplan-Meier estimator (48).
Results
Patient Characteristics
Between July 2016 and August 2019, blood samples of 88 consecutive patients with locally advanced, recurrent, or metastatic malignant disease were analyzed. No common infectious diseases were diagnosed. Data of 13 patients were excluded from the final analysis because of hematological malignancy 1), the lack of any biomarker data (2), death caused by rapid progression before the initiation of anticancer therapy (4), or by other cause of death than disease progression (6). Thus the final retrospective analysis included the data of 75 patients. The shortest censored survival time was 24 months, i.e., the time elapsed since July 2019. As of July 2021, six (8%) patients were still alive. Data of patient characteristics are described in Table 1. Additional data are given in Supplementary Tables S1–S11 in Supplementary File S1.
Baseline Biomarkers and Survival
The Kaplan-Meier plot was used to determine the median OS and the median follow-up times. With a median follow-up of 46.98 months [95% confidence interval (CI): 37.16–49.28] the median OS was 12.12 months (95% CI: 7.85–18.33) (Figure 1). Mean values of CRP, D-dimer, LDH, albumin, LMR, NLR, and PLR were 28.83 mg/L, 1.70 mcg/mL, 482.12 U/L, 41.62 g/L, 3.41, 4.29, and 168.83, respectively.
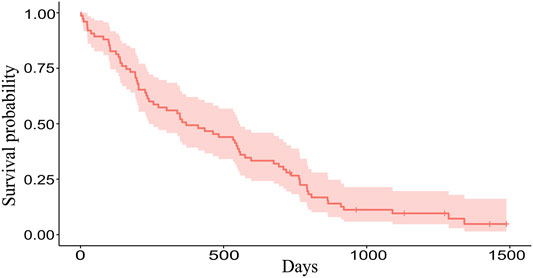
FIGURE 1. The Kaplan-Meier plot of 75 patients. Overall, 69 patients died and 6 patients are still alive (censored data). Median OS is 369 days (12.12 months), range 2–1488 days (0.06–48.89 months).
Determination of Cut-off Values
The following cut-off values were determined for CRP (Chi-squared = 20.85; p < 0.001), D-dimer (Chi-squared = 12.94; p < 0.001), LDH (Chi-squared = 10.45; p < 0.001), albumin (Chi-squared = 15.63; p < 0.001), LMR (Chi-squared = 3.45; p = 0.063), NLR (Chi-squared = 10.50; p < 0.001), and PLR (Chi-squared = 15.17; p < 0.001): 30.65 mg/L, 1.98 mcg/mL, 410.50 U/L, 44.35 g/L, 2.65, 4.34, and 168.20, respectively. The three most significant biomarkers were the following: CRP (Eta-squared = 0.188; large power size), albumin (Eta-squared = 0.147; large power size), and PLR (Eta-squared = 0.153; large power size).
The Relationship Between the Prognostic Cut-off Values and Survival
For each biomarker, a Kaplan-Meier plot was used to compare the median OS of the groups above and below the cut-off value (Figures 2A–C). For CRP and PLR (Figures 2A,C), longer survivals were found below than above the cut-off value. For albumin (Figure 2B), longer survival was found above the cut-off values (Table 2).
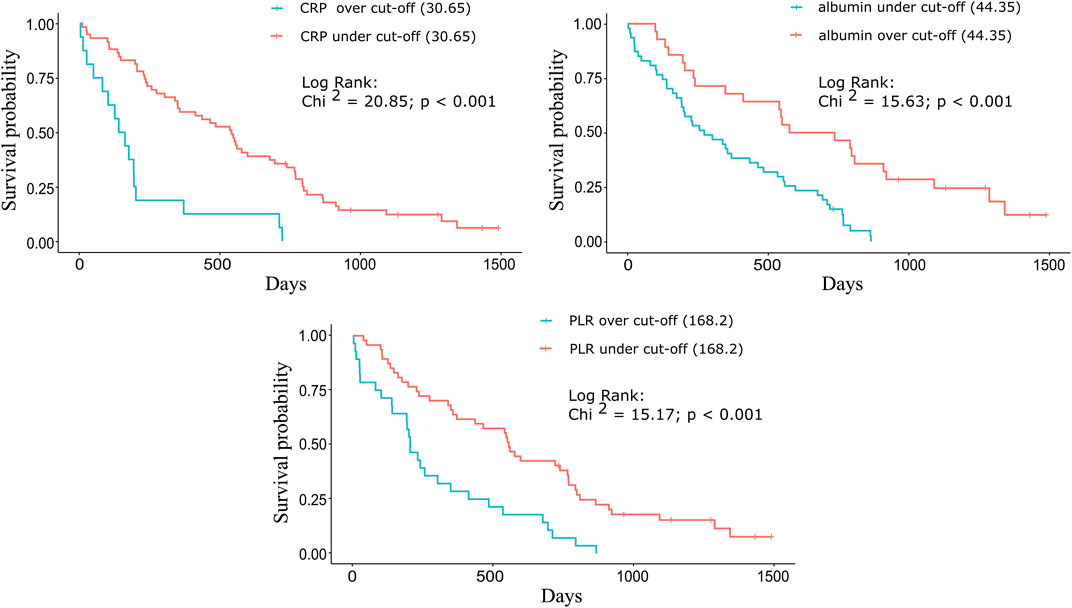
FIGURE 2. Kaplan-Meier plots for the three significant biomarkers. For (A) CRP and (C) PLR, longer survivals were found below the cut-off (30.65 mg/L and 168.20) values: 539 vs. 149 days (17.71 vs. 4.89 months) and 554 vs. 203 days (18.20 vs. 6.67 months). For (B) albumin, longer survival was found above the cut-off (44.35 g/L) value: 655.5 vs. 272 days (21.54 vs. 8.94 months).
Classification of Patients Into Risk Groups
With the combination of three biomarkers, prognostic groups were created independently from stage, histology, and time to progression on first line therapy (Supplementary File S1). Four prognostic groups were formed based on the cut-off values of each biomarker. Group 1: No biomarker with out-of-range value (ORV), defined by the cut-off value; group 2: One ORV biomarker; group 3: Two ORV biomarkers; and group 4: Three ORV biomarkers (Table 3). Significant differences were detected between these groups (Table 4, Figure 3). The likelihood ratio test of the Cox model regression parameters for the four groups was 29.5 (p < 0.001).

TABLE 3. The four prognostic groups based on the established cut-off values of the selected three biomarkers.
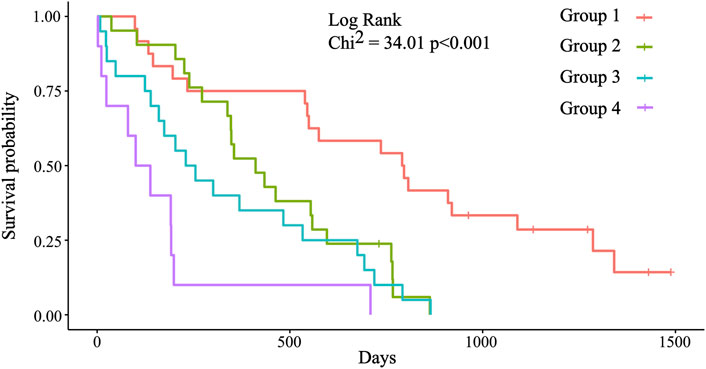
FIGURE 3. Kaplan-Meier survival plots for the four prognostic groups. Group 1: Median OS = 793.5 days (26.07 months); group 2: Median OS = 411.0 days (13.50 months); group 3: Median OS = 242.5 days (7.97 months); group 4: Median OS = 119 days (3.91 months). Significant differences were detected between group 1 (reference) and groups 2, 3, and 4 (p = 0.003; p < 0.001; p < 0.001).
Evaluation of the Survival Prediction of Three Biomarkers
We compared the median OS of groups with one ORV biomarker with that of groups with two and three ORV biomarkers using the Mann-Whitney test and Z statistic (Table 5). The comparison of the group of ORV albumin with the group of ORV CRP and albumin values indicated a significant difference (p = 0.04; Eta-squared = 0.067; medium power size). A similar significance was detected comparing the group ORV albumin with the group of ORV CRP and PLR (p = 0.026; Eta-squared = 0.087; medium power size). The cases in the groups of ORV CRP and PLR also had ORV albumin.
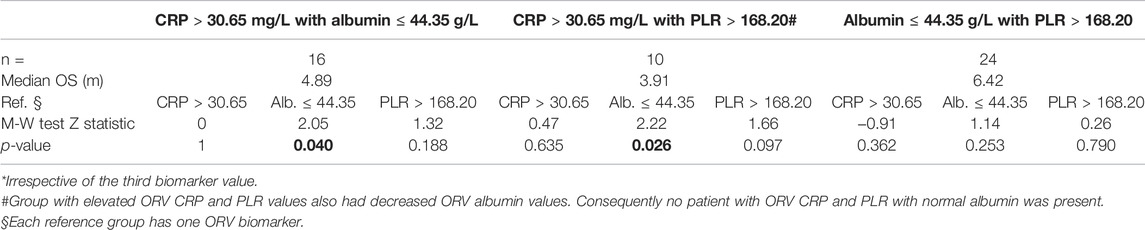
TABLE 5. Survival prediction of the usage of two ORV biomarkers* compared to the usage of one ORV biomarker.
No significant differences were found between the groups with two ORV biomarkers with three ORV biomarkers.
Discussion
In this retrospective and confirmatory analysis, we applied seven routinely measured clinical laboratory parameters (CRP, albumin, D-dimer, LDH, and based on CBC, calculated LMR, NLR and PLR) to a consecutive real-life patient population of locally advanced, recurrent, and metastatic malignant diseases at a single institution (Szent Lázár County Hospital), and searched for the most significant combination. These parameters and some of their combinations have already been proven to be independent prognostic factors for cancer.
Chronic low grade and intensity inflammation might precede malignant transformation and is considered to be a predisposing factor in cancer development (49). CRP is regarded as a biomarker of acute and chronic inflammation. Without other inflammatory processes, CRP may be increased (upper limit of normal CRP < 5 mg/L) in malignant diseases. In early-stage malignant diseases, a baseline normal CRP level correlates with longer OS. In locally advanced and metastatic settings, lower baseline CRP correlates with better prognosis (16).
Formation of serum albumin is determined by the osmotic colloid pressure, by the inflammatory and nutritional state of the body, and by hormonal factors. In cases of patients with localized malignant diseases both moderate hypoalbuminemia (<34 g/L) and a normal albumin level can occur. However, during disease progression, weight loss is accompanied by a significant decrease of albumin level. In a locally advanced and/or metastatic setting, serum albumin level diminishes independently in the presence of malnutrition. Lower baseline albumin suggests poor survival (19).
Elevated PLR (e.g., ≥200; >146.2; ≥180; >150; >220; >181.24) was proven to be an adverse prognostic factor in various cancers (34–45).
Here, the three most significant biomarkers were found: CRP, albumin, and PLR (Table 2), and stratification of the patients into one of the four groups was performed according to the number of ORV biomarkers (Table 3). We found that these prognostic groups enable the identification of good, moderate, intermediate, and poor OS patients with reasonable accuracy (Figure 3, Table 4). Based on our results, we can confirm that a combination of biomarkers probably has a better prognostic value than any of the single biomarkers (Table 5). Other prognostic threshold values published in previous studies were comparable to our results (16, 19, 34–45).
Our analysis has some limitations. First, the patient population for this small-scale retrospective analysis is histologically heterogenous. Second, regarding the stage, these unbalanced cohorts of locally advanced, recurrent, or metastatic diseases are also heterogenous. Third, the identified cut-off values by this study for CRP, albumin, and PLR are slightly different from those used by other studies, therefore they need to be validated in a large-scale prospective study. Fourth, there are multiple factors that could have a possible influence on the OS of patients that were not monitored in our analysis.
Conclusion
Based on our analysis, we can confirm that the combination of serum biomarkers measured at baseline would provide accurate estimation for OS in real-life advanced cancer patients. We were able to establish consistent prognostic groups using the most significant three biomarkers. The OS was significantly different in each of the prognostic groups developed. One advantage of our study is that these parameters can be routinely measured without additional costs. We are persuaded that the prognostic significance of these and other biomarker patterns, and their role in relation to the well-established prognostic systems, warrants further investigation and validation in large prospective cohorts of real-life cancer patients.
Data Availability Statement
The datasets presented in this study can be found in online repositories. The names of the repository/repositories and accession number(s) can be found in the article/Supplementary Material.
Ethics Statement
Ethical approval was waived by the Medical Research Council (No. IV/5406- 1 /2021/EKU) in view of the retrospective nature of the study and all the procedures being performed were part of the routine care.
Author Contributions
DD collected the data and summarized them in a datasheet, wrote the manuscript, and created the tables. SK performed all statistical tests and drew the statistical figures. AT raised the study idea, supported the study, and critically reviewed the manuscript.
Conflict of Interest
The authors declare that the research was conducted in the absence of any commercial or financial relationships that could be construed as a potential conflict of interest.
Acknowledgments
Hereby the authors of this work thank the detailed evaluations and the valuable suggestions of Prof. Barna Vásárhelyi, the Director of Department of Laboratory Medicine of the Semmelweis University, Budapest.
Supplementary Material
The Supplementary Material for this article can be found online at: https://www.por-journal.com/articles/10.3389/pore.2022.1610004/full#supplementary-material
Supplementary File S1 | Summary of the data of 13 excluded patients. Additional data of 75 patients demonstrated in Supplementary Tables S1–S11.
Supplementary File S2 | The biomarker values in a tab separated value file for “Cutoff Finder.”
Supplementary File S3 | The biomarker and survival values in semicolon separated value file for the analysis inR Studio Software.
Supplementary File S4 | R‐code is extended with the script of the KM curves for the prognostic groups.
References
1. Nixon, AB, Pang, H, Starr, MD, Friedman, PN, Bertagnolli, MM, Kindler, HL, et al. Prognostic and Predictive Blood-Based Biomarkers in Patients with Advanced Pancreatic Cancer: Results from CALGB80303 (Alliance). Clin Cancer Res (2013) 19:6957–66. doi:10.1158/1078-0432.CCR-13-0926
2. Nakagawa, K, Tanaka, K, Nojiri, K, Kumamoto, T, Takeda, K, Ueda, M, et al. The Modified Glasgow Prognostic Score as a Predictor of Survival after Hepatectomy for Colorectal Liver Metastases. Ann Surg Oncol (2014) 21:1711–8. doi:10.1245/s10434-013-3342-6
3. Liao, DW, Hu, X, Wang, Y, Yang, ZQ, and Li, X. C-reactive Protein Is a Predictor of Prognosis of Prostate Cancer: A Systematic Review and Meta-Analysis. Ann Clin Lab Sci (2020) 50:161–71.
4. Chen, Y, Cong, R, Ji, C, and Ruan, W. The Prognostic Role of C‐reactive Protein in Patients with Head and Neck Squamous Cell Carcinoma: A Meta‐analysis. Cancer Med (2020) 9:9541–53. doi:10.1002/cam4.3520
5. Wang, Y, and Wang, Z. Predictive Value of Plasma D-Dimer Levels in Patients with Advanced Non-small-cell Lung Cancer. Ott (2015) 8:805–8. doi:10.2147/OTT.S78154
6. Liu, P, Zhu, y., and Liu, l. Elevated Pretreatment Plasma D-Dimer Levels And platelet Counts Predict Poor Prognosis In pancreatic Adenocarcinoma. Ott (2015) 8:1335–40. doi:10.2147/OTT.S82329
7. Wu, J, Fu, Z, Liu, G, Xu, P, Xu, J, and Jia, X. Clinical Significance of Plasma D-Dimer in Ovarian Cancer. Medicine (Baltimore) (2017) 96:e7062. doi:10.1097/MD.0000000000007062
8. Ma, M, Cao, R, Wang, W, Wang, B, Yang, Y, Huang, Y, et al. The D-Dimer Level Predicts the Prognosis in Patients with Lung Cancer: a Systematic Review and Meta-Analysis. J Cardiothorac Surg (2021) 16:243. doi:10.1186/s13019-021-01618-4
9. Ji, F, Fu, S-J, Guo, Z-Y, Pang, H, Ju, W-Q, Wang, D-P, et al. Prognostic Value of Combined Preoperative Lactate Dehydrogenase and Alkaline Phosphatase Levels in Patients with Resectable Pancreatic Ductal Adenocarcinoma. Medicine (Baltimore) (2016) 95:e4065. doi:10.1097/MD.0000000000004065
10. Liu, R, Cao, J, Gao, X, Zhang, J, Wang, L, Wang, B, et al. Overall Survival of Cancer Patients with Serum Lactate Dehydrogenase Greater Than 1000 IU/L. Tumor Biol (2016) 37:14083–8. doi:10.1007/s13277-016-5228-2
11. Li, F, Xiang, H, Pang, Z, Chen, Z, Dai, J, Chen, S, et al. Association between Lactate Dehydrogenase Levels and Oncologic Outcomes in Metastatic Prostate Cancer: A Meta‐analysis. Cancer Med (2020) 9:7341–51. doi:10.1002/cam4.3108
12. Deng, T, Zhang, J, Meng, Y, Zhou, Y, and Li, W. Higher Pretreatment Lactate Dehydrogenase Concentration Predicts Worse Overall Survival in Patients with Lung Cancer. Medicine (Baltimore) (2018) 97:e12524. doi:10.1097/MD.0000000000012524
13. Win, T, Sharples, L, Groves, AM, Ritchie, AJ, Wells, FC, and Laroche, CM. Predicting Survival in Potentially Curable Lung Cancer Patients. Lung (2008) 186:97–102. doi:10.1007/s00408-007-9067-1
14. Shibutani, M, Maeda, K, Nagahara, H, Iseki, Y, Ikeya, T, and Hirakawa, K. Prognostic Significance of the Preoperative Ratio of C-Reactive Protein to Albumin in Patients with Colorectal Cancer. Anticancer Res (2016) 36:995–1001.
15. Liu, J, Wang, F, Li, S, Huang, W, Jia, Y, and Wei, C. The Prognostic Significance of Preoperative Serum Albumin in Urothelial Carcinoma: a Systematic Review and Meta-Analysis. Biosci Rep (2018) 38:BSR20180214. doi:10.1042/BSR20180214
16. Deme, D, and Telekes, A. A C-Reaktív Protein (CRP) Plazmaszintjének Prognosztikai Jelentősége Az Onkológiában. Orvosi Hetilap (2017) 158:243–56. Hungarian. doi:10.1556/650.2017.30646
17. Deme, D, and Telekes, A. Prognostic Importance of Cross-Linked Fibrin Degradation Products (D-Dimer) in Oncology. Magy Onkol (2017) 61:319–26. Hungarian.
18. Deme, D, and Telekes, A. A Laktátdehidrogenáz (LDH) Prognosztikai Jelentősége Az Onkológiában. Orvosi Hetilap (2017) 158:1977–88. Hungarian. doi:10.1556/650.2017.30890
19. Deme, D, and Telekes, A. Az Albumin Prognosztikai Jelentősége Az Onkológiában. Orvosi Hetilap (2018) 159:96–106. Hungarian. doi:10.1556/650.2018.30885
20. Song, L, Zhu, J, Li, Z, Wei, T, Gong, R, and Lei, J. The Prognostic Value of the Lymphocyte-To-Monocyte Ratio for High-Risk Papillary Thyroid Carcinoma. Cmar (2019) Vol. 11:8451–62. doi:10.2147/CMAR.S219163
21. Chen, X-Q, Xue, C-R, Hou, P, Lin, B-Q, and Zhang, J-R. Lymphocyte-to-monocyte Ratio Effectively Predicts Survival Outcome of Patients with Obstructive Colorectal Cancer. Wjg (2019) 25:4970–84. doi:10.3748/wjg.v25.i33.4970
22. Song, Q, Wu, J-z., and Wang, S. Low Preoperative Lymphocyte to Monocyte Ratio Serves as a Worse Prognostic Marker in Patients with Esophageal Squamous Cell Carcinoma Undergoing Curative Tumor Resection. J Cancer (2019) 10:2057–62. doi:10.7150/jca.29383
23. Lu, C, Zhou, L, Ouyang, J, and Yang, H. Prognostic Value of Lymphocyte-To-Monocyte Ratio in Ovarian Cancer. Medicine (Baltimore) (2019) 98:e15876. doi:10.1097/MD.0000000000015876
24. Zhou, W, Kuang, T, Han, X, Chen, W, Xu, X, Lou, W, et al. Prognostic Role of Lymphocyte-To-Monocyte Ratio in Pancreatic Neuroendocrine Neoplasms. Endocr Connect (2020) 9:289–98. pii: EC-19-0541.R1. doi:10.1530/EC-19-0541
25. Templeton, AJ, McNamara, MG, Šeruga, B, Vera-Badillo, FE, Aneja, P, Ocaña, A, et al. Prognostic Role of Neutrophil-To-Lymphocyte Ratio in Solid Tumors: a Systematic Review and Meta-Analysis. J Natl Cancer Inst (2014) 106(6):dju124. doi:10.1093/jnci/dju124
26. Yang, Y, Liu, R, Ren, F, Guo, R, and Zhang, P. Prognostic and Clinicopathological Significance of Neutrophil-To-Lymphocyte Ratio in Patients with Oral Cancer. Biosci Rep (2018) 38:BSR20181550. pii. doi:10.1042/BSR20181550
27. Chen, G, Zhu, L, Yang, Y, Long, Y, Li, X, and Wang, Y. Prognostic Role of Neutrophil to Lymphocyte Ratio in Ovarian Cancer: A Meta-Analysis. Technol Cancer Res Treat (2018) 17:153303381879150. doi:10.1177/1533033818791500
28. Liu, G, Ke, L-c., and Sun, S-R. Prognostic Value of Pretreatment Neutrophil-To-Lymphocyte Ratio in Patients with Soft Tissue Sarcoma. Medicine (Baltimore) (2018) 97:e12176. doi:10.1097/MD.0000000000012176
29. Pirozzolo, G, Gisbertz, SS, Castoro, C, van Berge Henegouwen, MI, and Scarpa, M. Neutrophil-to-lymphocyte Ratio as Prognostic Marker in Esophageal Cancer: a Systematic Review and Meta-Analysis. J Thorac Dis (2019) 11:3136–45. doi:10.21037/jtd.2019.07.30
30. Wang, Z, Zhan, P, Lv, Y, Shen, K, Wei, Y, Liu, H, et al. Prognostic Role of Pretreatment Neutrophil-To-Lymphocyte Ratio in Non-small Cell Lung Cancer Patients Treated with Systemic Therapy: a Meta-Analysis. Transl Lung Cancer Res (2019) 8:214–26. doi:10.21037/tlcr.2019.06.10
31. Ding, Y, Zhang, S, and Qiao, J. Prognostic Value of Neutrophil-To-Lymphocyte Ratio in Melanoma. Medicine (Baltimore) (2018) 97:e11446. doi:10.1097/MD.0000000000011446
33. Cho, J-K, Kim, MW, Choi, IS, Moon, UY, Kim, M-J, Sohn, I, et al. Optimal Cutoff of Pretreatment Neutrophil-To-Lymphocyte Ratio in Head and Neck Cancer Patients: a Meta-Analysis and Validation Study. BMC Cancer (2018) 18:969. doi:10.1186/s12885-018-4876-6
34. Tian, C, Song, W, Tian, X, and Sun, Y. Prognostic Significance of Platelet-To-Lymphocyte Ratio in Patients with Ovarian Cancer: A Meta-Analysis. Eur J Clin Invest (2018) 48(5):e12917. doi:10.1111/eci.12917
35. Wang, J, Zhou, X, He, Y, Chen, X, Liu, N, Ding, Z, et al. Prognostic Role of Platelet to Lymphocyte Ratio in Prostate Cancer. Medicine (Baltimore) (2018) 97(40):e12504. doi:10.1097/MD.0000000000012504
36. Cao, W, Yao, X, Cen, D, Zhi, Y, Zhu, N, and Xu, L. The Prognostic Role of Platelet-To-Lymphocyte Ratio on Overall Survival in Gastric Cancer: a Systematic Review and Meta-Analysis. BMC Gastroenterol (2020) 20:16. doi:10.1186/s12876-020-1167-x
37. Bardash, Y, Olson, C, Herman, W, Khaymovich, J, Costantino, P, and Tham, T. Platelet-Lymphocyte Ratio as a Predictor of Prognosis in Head and Neck Cancer: A Systematic Review and Meta-Analysis. Oncol Res Treat (2019) 42:665–77. doi:10.1159/000502750
38. Wang, X, Ni, X, and Tang, G. Prognostic Role of Platelet-To-Lymphocyte Ratio in Patients with Bladder Cancer: A Meta-Analysis. Front Oncol (2019) 9:757. doi:10.3389/fonc.2019.00757
39. Zhang, M, Huang, X-z., Song, Y-x., Gao, P, Sun, J-x., and Wang, Z-N. High Platelet-To-Lymphocyte Ratio Predicts Poor Prognosis and Clinicopathological Characteristics in Patients with Breast Cancer: A Meta-Analysis. Biomed Res Int (2017) 2017:1–11. Article ID 9503025. doi:10.1155/2017/9503025
40. Xu, W, Wang, W, Yang, M, Song, L, Xiong, J, Lin, J, et al. Prognostic Significance of the Platelet-To-Lymphocyte Ratio in Ovarian Cancer: a Meta-Analysis. Translational Cancer Res (2018) 7:552–60. doi:10.21037/21627
41. Song, W, Tian, C, Wang, K, Zhang, R-J, and Zou, S-B. Preoperative Platelet Lymphocyte Ratio as Independent Predictors of Prognosis in Pancreatic Cancer: A Systematic Review and Meta-Analysis. PLOS ONE (2017) 12:e0178762. doi:10.1371/journal.pone.0178762
42. You, J, Zhu, G-Q, Xie, L, Liu, W-Y, Shi, L, Wang, O-C, et al. Preoperative Platelet to Lymphocyte Ratio Is a Valuable Prognostic Biomarker in Patients with Colorectal Cancer. Oncotarget (2016) 7:25516–27. doi:10.18632/oncotarget.8334
43. Zhang, X, Wang, Y, Zhao, L, Sang, S, and Zhang, L. Prognostic Value of Platelet-To-Lymphocyte Ratio in Oncologic Outcomes of Esophageal Cancer: A Systematic Review and Meta-Analysis. Int J Biol Markers (2018) 33:335–44. doi:10.1177/1724600818766889
44. Lim, JU, Yeo, CD, Kang, HS, Park, CK, Kim, JS, Kim, JW, et al. Elevated Pretreatment Platelet-To-Lymphocyte Ratio Is Associated with Poor Survival in Stage IV Non-small Cell Lung Cancer with Malignant Pleural Effusion. Sci Rep (2019) 9:4721. doi:10.1038/s41598-019-41289-9
45. Lee, Y, Kim, YW, Park, DK, and Hwang, IC. Inverse Association between Platelet-Lymphocyte Ratio and Prognosis in Terminally Ill Cancer Patients: A Preliminary Study. J Palliat Med (2017) 20:533–7. doi:10.1089/jpm.2016.0338
46. Budczies, J, Klauschen, F, Sinn, BV, Győrffy, B, Schmitt, WD, Darb-Esfahani, S, et al. Cutoff Finder: a Comprehensive and Straightforward Web Application Enabling Rapid Biomarker Cutoff Optimization. PLoS One (2012) 7:e51862. Online Cutoff Finder tool is available at https://molpathoheidelberg.shinyapps.io/CutoffFinder_v1/ (Accessed September 18, 2021). doi:10.1371/journal.pone.0051862
47.R Core Team. R: A Language and Environment for Statistical Computing. Vienna: R Foundation for Statistical Computing (2019). Available at: https://www.R-project.org. (Accessed July 11, 2021).
48. Shuster, JJ. Median Follow-Up in Clinical Trials. Jco (1991) 9:191–2. doi:10.1200/JCO.1991.9.1.191
Keywords: overall survival, advanced cancer, serum biomarkers, prognostic importance, CRP, albumin, PLR
Citation: Deme D, Kovacs S and Telekes A (2022) Overall Survival Prediction of Advanced Cancer Patients by Selection of the Most Significant Baseline Serum Biomarker Combination. Pathol. Oncol. Res. 28:1610004. doi: 10.3389/pore.2022.1610004
Received: 04 August 2021; Accepted: 04 January 2022;
Published: 31 January 2022.
Edited and reviewed by:
Anna Sebestyén, Semmelweis University, HungaryCopyright © 2022 Deme, Kovacs and Telekes. This is an open-access article distributed under the terms of the Creative Commons Attribution License (CC BY). The use, distribution or reproduction in other forums is permitted, provided the original author(s) and the copyright owner(s) are credited and that the original publication in this journal is cited, in accordance with accepted academic practice. No use, distribution or reproduction is permitted which does not comply with these terms.
*Correspondence: Daniel Deme, ZGFuaWVsZGVtZV9tZEB5bWFpbC5jb20=