- 1Department of Integrated Research Center for Genome Polymorphism, The Catholic University of Korea, Seoul, Korea
- 2Department of Microbiology, The Catholic University of Korea, Seoul, Korea
- 3Department of Cancer Research Center, The Catholic University of Korea, Seoul, Korea
- 4Department of Gynecology/Obstetrics, The Catholic University of Korea, Seoul, Korea
- 5Department of Biochemistry, The Catholic University of Korea, Seoul, Korea
- 6Department of Pathology, The Catholic University of Korea, Seoul, Korea
Teratoma is a type of germ cell tumor that originates from totipotential germ cells that are present in gonads, which can differentiate into any of the cell types found in adult tissues. Ovarian teratomas are usually mature cystic teratomas (OMCTs, also known as dermoid cysts). Chromosome studies in OMCTs show that the chromosomes are uniformly homozygous with karyotype of 46, XX, indicating that they may be parthenogenic tumors that arise from a single ovum after thefirst meiotic division. However, the tissues in OMCTs have been known to be morphologically and immunophenotypically identical to the orthotopic tissues. Currently, expression profiles of tissue components in OMCTs are not known. To identify whether OMCT tissues are expressionally similar to or different from the orthotopic tissues, we adopted single-cell RNA-sequencing (scRNA-seq), and analyzed transcriptomes of individual cells in heterogenous tissues of two OMCTs. We found that transcriptome profiles of the OMCTs at single cell level were not significantly different from those of normal cells in orthotopic locations. The present data suggest that parthenogeneticlly altered OMCTs may not alter expression profiles of inrivirual tissue components in OMCTs.
Introduction
Teratomas are germ cell tumors commonly composed of multiple cell types derived from one or more of the three germ layers and, in many series, the most common excised ovarian tumor [1, 2]. Ovarian teratomas are usually mature cystic teratomas (OMCTs, also known as dermoid cysts), which account for about 11% of all ovarian tumors [1, 2]. In OMCTs, ectodermal derivatives, including epidermis and hair follicles are most prominent, but mesodermal and endodermal derivatives are also found mixedly together [1, 2]. These tissues are known to be histologically identical to the orthotopic tissues. OMCTs are diploid, and cytogenetic study demonstrates that they almost always have 46, XX karyotype [3–5]. Also, they are usually homozygous, indicating that they derive most often from a ovum (parthenogenic) that has completed meiosis I but not meiosis II [3–5]. OMCTs harbor distinct methylation profiles of imprinted genes with high and low levels of methylation for maternally and paternally imprinted genes, respectively, supporting the parthenogenic origin [6].
In addition to the parthenogenic alteration, there exists evidence that suggests epigenetic alterations in OMCTs. For example, there is expressional difference in microRNAs between OMCTs and normal ovarian tissues [7]. OMCTs of both mice and human srongly express transcriptional regularor HDAC1, which can be a novel marker for benign teratomas [8]. To our knowledge, transcription profiles of OMCTs have not yet been repprted probably due to the technological limitations with the heterogenous tissue components, which would lead to averaging of expression data. Single-cell RNA-sequencing (scRNA-seq) allows researchers to obtain transcriptome of individual cells, which might further identify population of heterogenous cells by avoiding expression data averaging [9]. In this study, we studied two OMCTs by scRNA-seq that analyzed transcriptomes on a cell-by-cell basis with next-generation sequencing (NGS) cDNA library.
Materials and Methods
Tissue Isolation
Ovarian cystectomy specimens were collected from two patients (26 year-old and 48 year-old females) pathologically confirmed as mature cystic teratoma (OMCT). They were separately minced into fragments and digested with collagenase/dispase (Roche Diagnostics, Mannheim, Germany) and DNase I (Roche Diagnostics) for 30 min at 37°C with agitation. The dissociated cell suspension was filtered through 70 μm strainer, washed with phosphate-buffered saline, and centrifuged at x400g for 5 min. The cell pellet was resuspended in RPMI 1640 medium for further use.
Single-Cell Library Preparation, Sequencing and Pre-processing
Chromium Single Cell 3′ v3 (10x Genomics, Pleasanton, CA) library preparation was performed according to the manufacturer’s protocol. Each library from the two OMCTs was separately sequenced on the Illumina Hiseq platform (Illumina, San Diego, CA) to achieve around 70,000 reads per cell. FASTQ files were processed using Cell Ranger 3.1.0 (10x Genomics) analysis pipeline and were applied to generate a digital gene-cell matrix. Briefly, the files were aligned to the human GRCh38 reference genome followed by unique molecular identifier (UMI) and barcode counting, constructing the UMI count matrices.
Quality Control and Clustering Analysis
Raw UMI-counts were further analyzed using Seurat R package version 3.1.5 [10]. Briefly, cells with fewer than 200 genes, more than 7,000 genes, or more than 20% mitochondria content were excluded for each sample. Filtered gene-barcode matrices of the two samples were integrated to remove batch effects across different patients using Seurat FindIntegrationAnchors and IntegrateData function [10]. Then uniform manifold approximation and projection (UMAP) was performed on the top 30 principal components for visualizing the cells. Meanwhile, graph-based clustering was performed on the PCA-reduced data for clustering analysis. The resolution was set to 0.9 to obtain a finer result. Finally, the Wilcoxon rank sum test was used to identify differentially expressed genes (DEGs) in each cluster with those in all other clusters using Seurat FindAllMarkers function. Additionally, gene ontology analysis was performed with the top 50 DEGs of each cluster using MSigDB [11] to investigate the functional profiles for genes and gene clusters. We used the SingleR [12] and the Gene Expression Deconvolution Interactive Tool (GEDIT) (http://webtools.mcdb.ucla.edu/) to annotate the clusters and predict the cell type composition.
Results
The scRNA-seq identified a total of 21,652 expressed genes in 8,900 cells from two OMCTs (a median of 1,659 genes per cell). We were able to define different cell populations constituting the OMCTs using UMAP and unsupervized clustering (Figure 1A), which showed 27 distinct clusters of immune cells (PTPRC, CXCR4), stromal cells/fibroblasts (DCN, PDGFRA), endothelial cells (VWF, CDH5), epithelial cells/keratinocytes (KRT18, KRT14) and melanocytes (PMEL, MLANA) (Figures 1B,C). Immune cells included T cells, B cells and macrophages according to CD3D, IGKC, and AIF1 expressions, respectively (Supplementary Figure S1).
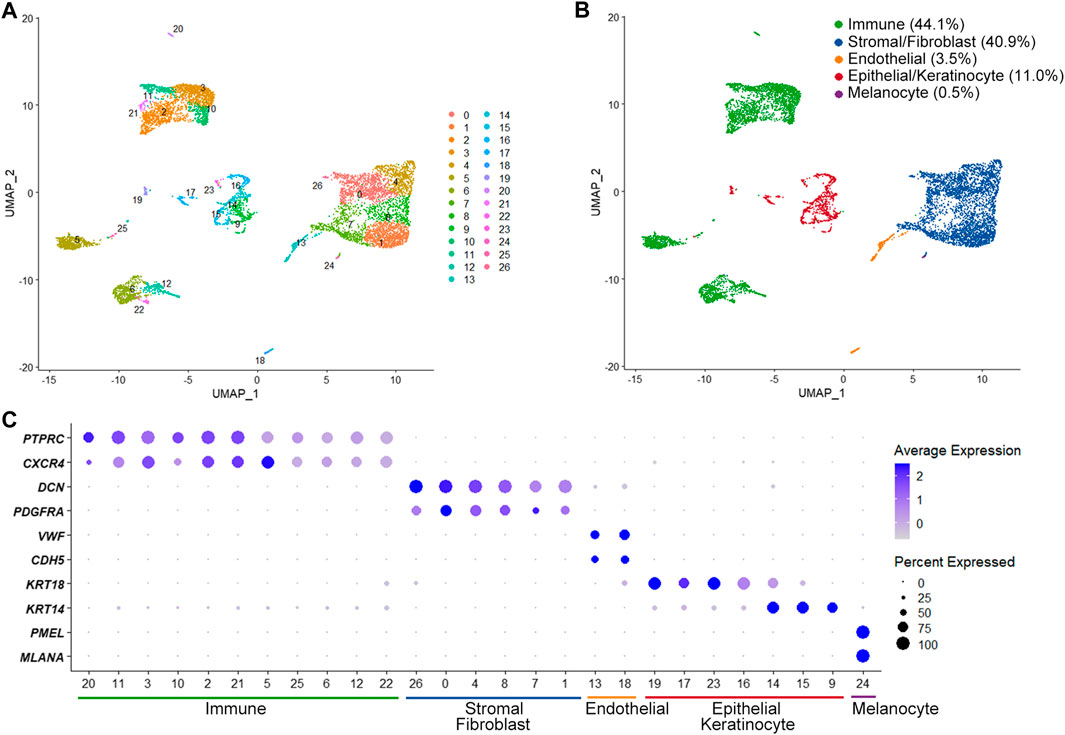
FIGURE 1. Single-cell transcriptome analysis of ovarian mature cystic teratomas. (A) Unsupervized clustering from merged scRNA-seq data of two ovarian mature cystic teratomas. Each dot represents an individual cell, colored according to each cluster. (B) Main cell clusters identified with the cell type-specific marker genes. (C) Dot plot showing expression of lineage-specific marker genes. The color and size of each dot represents the average gene expression value and the proportion of expressed cells of a given gene in each cluster.
Epithelial cell clusters (cluster 9, 14, 15, 16, 17, 19, 23) were further divided according to the expression levels of keratin isoforms and their DEGs (Figures 2A–C and Supplementary Figure S2). They were largely discriminated by the expression of KRT7 and KRT19 in simple ductal epithelia and of KRT5 and KRT14 in stratified epithelia [13] (Figure 2A). In detail, the cluster 19 with elevated expression of secretory genes MUCL1 and PIP, lipid metabolism genes GLYATL2, SERHL2, and UGT2B28 and holocrine secretion gene MGST1 [14] was consistent with lipid-producing sebaceous gland cells (Figures 2B,C and Supplementary Figure S2). Similarly, the clusters 17, 23, 16, 14 and 15 were defined sweat gland cells, lung secretory cells, mucin-producing ductal cells, mixed/epidermal differentiating keratinocyte and basal keratinocytes, respectively, indicating multi-organ nature of OMCT epithelial cell compositions (Figures 2B,C and Supplementary Figure S2). The GO enrichment analysis of the gene signatures also supported the association of markers with relevant cell types and processes (Figure 2D). Expression status of the epithelial cell clusters were well matched with those of reference datasets [15–17] for normal cell types (Supplementary Figure S3A). When compared to the Skin Signatures database [18], transcription signatures of the clusters in OMCTs were well matched with specific skin cells (Supplementary Figure S3B). Six fibroblasts/stromal cell clusters were largely categorized into fibroblasts (clusters 0, 4, 26) and ovarian stromal cells (clusters 1, 7, 8) based on their respective expression of COL1A1/COL1A2 and steroidogenesis-related genes STAR and FHL2 (Figure 2E). The cluster 26 expressed dermal papillary fibroblast-related genes PTGDS and MGP (Figure 2F and Supplementary Figure S4). The clusters 0 and 4 expressed chemokines CXCL12 and CCL2, and complement genes C3 and CFD, consistent with pro-inflammatory fibroblasts (Figure 2F and Supplementary Figure S4). The clusters 1, 7 and 8 highly expressed steroidogenic genes STAR as well as tissue remodeling genes HAS1, ADAMTS1 and ADAMTS4, consistent with theca-stromal cells from ovary [9] (Figure 2F and Supplementary Figure S4), which might be included from non-tumor tissues from ovaries. The fibroblast clusters were enriched for collagen containing extracellular matrix, whereas the stromal cell clusters were enriched for response to lipid, endogenous stimulus or hormone in GO analysis (Figure 2G).
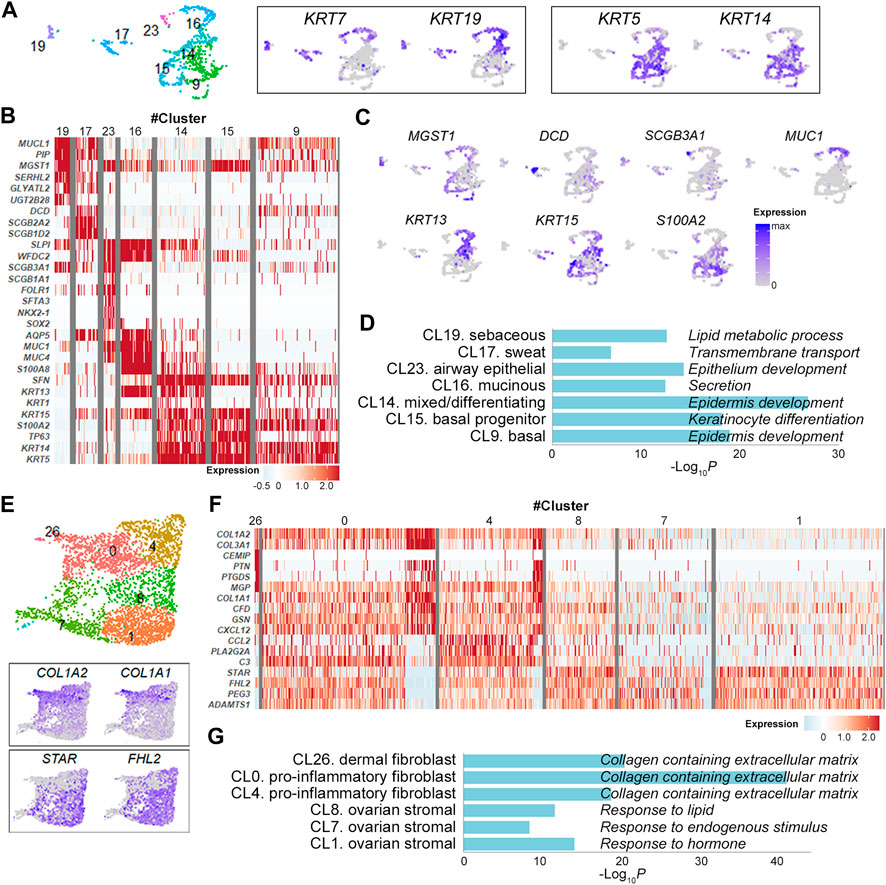
FIGURE 2. Identification of epithelial cell and fibroblast/stromal cell clusters. (A) Epithelial cell clusters with the relative expression of keratin isoform on the UMAP plot. Each dot represents an individual cell. (B) Heatmap showing representative marker genes for each epithelial cell cluster. (C) UMAP plots showing the relative expression of selected marker genes from each epithelial cell cluster. (D) Gene ontology analysis of epithelial cell clusters. The graph shows p values for the most significant ontology term for each cluster. (E) Fibroblast/stromal cell clusters with the relative expression of selected marker genes on the UMAP plot. (F) Heatmap showing representative marker genes for each fibroblast/stromal cell cluster. (G) Gene ontology analysis of fibroblast/stromal cell clusters. The graph shows p values for the most significant ontology term for each cluster.
Discussion
The OMCT is a distinct tumor in which heterogenous tissues mimicking mature multi-organ tissues with predominant skin components [1, 2]. The aim of this study was to address transcription profiles of heterogenous cell types of OMCTs, which had not been identified. For this, we adopted the scRNA-seq and found that transcriptomes of individual cell types in OMCTs were not different from those of normal cells in orthotopic locations. Although the OMCT arises from an ovum without a sperm (parthenogenic), our data indicate that the uniparental chromosomes may not affect the expression of individual tissues in OMCTs. The parthenogenesis might lead the ovum to an OMCT instead of leading to embryo development. The limitation of our study was to analyze only two cases. Although OMCT is a benign tumor with relatively uniform clinical features, analysis of a larger cohort will be needed to solidify our results.
The scRNA-seq is a high-resolution assay used to interrogate transcriptome of individual cells within tissues that may help find novel discoveries in tissue heterogeneity [9, 19, 20]. For example, in cancer, scRNA-seq of individual cells may give insight into the existence and behavior of different cell types in both tumor and microenvironment cells [19, 20]. The scRNA-seq is becoming widely used across biological field including developmental biology, oncology, immunology and Infectious diseases [20]. In onclogy, scRNA-seq analyzed diverse cancer types including cancers of breast, colon and ovary. The scRNA-seq for serous ovarian carcinomas identified heterogenous expression profiles of cancer cells as well as ascitic inflammatory cells that might alter disease progression and treatment responses [19]. In this study, we for the first time disclosed the transcriptome profiles of OMCTs at a single cell level and found that they may recapitulate expressions of normal counterparts. Our study could be an example to analyze other gynecologic diseases with heterogenous tissues such as other germ cell tumors using scRNA-seq.
Data Availability Statement
The datasets presented in this study can be found in online repositories. The names of the repository/repositories and accession number(s) can be found in the article/Supplementary Material.
Ethics Statement
This study was approved by the ethics committee of Seoul Saint Mary Hospital, and all patients provided written informed consent. Patient identity protection was maintained throughout the study.
Author Contributions
Concept and design: SL and YC; experimants, data analysis and interpretation: SS, S-HJ, and Y-JC; manuscript drafting and approval of the final version: all authors; study supervision: SL and YC.
Funding
This study was supported by grants from Korea Research Foundation (2019R1A5A2027588, 2020R1A2C2005031 and 2017R1E1A1A01074913).
Conflict of Interest
The authors declare that the research was conducted in the absence of any commercial or financial relationships that could be construed as a potential conflict of interest.
Supplementary Material
The Supplementary Material for this article can be found online at: https://www.por-journal.com/articles/10.3389/pore.2021.604228/full#supplementary-material.
References
1. Peterson, WF, Prevost, EC, Edmunds, FT, Hundley, JM, and Morris, FK. Benign cystic teratomas of the ovary. Am J Obstet Gynecol (1955). 70:368–82. doi:10.1016/s0002-9378(16)37681-5
2. Ayhan, A, Bukulmez, O, Genc, C, Karamursel, BS, and Ayhan, A. Mature cystic teratomas of the ovary: case series from one institution over 34 years. Eur J Obstet Gynecol Reprod Biol (2000). 88:153–7. doi:10.1016/s0301-2115(99)00141-4
3. Linder, D, McCaw, BK, and Hecht, F. Parthenogenic origin of benign ovarian teratomas. N Engl J Med (1975). 292:63–6. doi:10.1056/nejm197501092920202
4. Parrington, JM, West, LF, and Povey, S. The origin of ovarian teratomas. J Med Genet (1984). 21:4–12. doi:10.1136/jmg.21.1.4
5. Surti, U, Hoffner, L, Chakravarti, A, and Ferrell, RE. Genetics and biology of human ovarian teratomas. I. Cytogenetic analysis and mechanism of origin. Am J Hum Genet (1990). 47:635–43.
6. Kato, N, Kamataki, A, and Kurotaki, H. Methylation profiles of imprinted genes are distinct between mature ovarian teratoma, complete hydatidiform mole, and extragonadal mature teratoma. Mod Pathol, 34, 502 (2021). doi:10.1038/s41379-020-00668-8
7. Ding, Y, Gu, X-Y, Xu, F, Shi, X-Y, Yang, D-Z, Zhong, J, et al. MicroRNA expression profiling of mature ovarian teratomas. Oncol Lett (2012). 3:35–8. doi:10.3892/ol.2011.438
8. Simboeck, E, and Di Croce, L HDAC1, a novel marker for benign teratomas. EMBO J (2010). 29:3893–5. doi:10.1038/emboj.2010.281
9. Wagner, M, Yoshihara, M, Douagi, I, Damdimopoulos, A, Panula, S, Petropoulos, S, et al. Single-cell analysis of human ovarian cortex identifies distinct cell populations but no oogonial stem cells. Nat Commun (2020). 11:1147. doi:10.1038/s41467-020-14936-3
10. Stuart, T, Butler, A, Hoffman, P, Hafemeister, C, Papalexi, E, Mauck, WM, et al. Comprehensive integration of single-cell data. Cell (2019). 177:1888–902. doi:10.1016/j.cell.2019.05.031
11. Liberzon, A, Birger, C, Thorvaldsdóttir, H, Ghandi, M, Mesirov, JP, and Tamayo, P. The molecular signatures database hallmark gene set collection. Cell Syst (2015). 1:417–25. doi:10.1016/j.cels.2015.12.004
12. Aran, D, Looney, AP, Liu, L, Wu, E, Fong, V, Hsu, A, et al. Reference-based analysis of lung single-cell sequencing reveals a transitional profibrotic macrophage. Nat Immunol (2019). 20:163–72. doi:10.1038/s41590-018-0276-y
13. Chu, PG, and Weiss, LM. Keratin expression in human tissues and neoplasms. Histopathology (2002). 40:403–39. doi:10.1046/j.1365-2559.2002.01387.x
14. Cheng, JB, Sedgewick, AJ, Finnegan, AI, Harirchian, P, Lee, J, Kwon, S, et al. Transcriptional programming of normal and inflamed human epidermis at single-cell resolution. Cell Rep (2018). 25:871–83. doi:10.1016/j.celrep.2018.09.006
15.The ENCODE Project Consortium. An integrated encyclopedia of DNA elements in the human genome. Nature (2012). 489:57–74. doi:10.1038/nature11247
16. Martens, JHA, and Stunnenberg, HG. BLUEPRINT: mapping human blood cell epigenomes. Haematologica (2013). 98:1487–9. doi:10.3324/haematol.2013.094243
17. Mabbott, NA, Baillie, J, Brown, H, Freeman, TC, and Hume, DA. An expression atlas of human primary cells: inference of gene function from coexpression networks. BMC Genomics (2013). 14:632. doi:10.1186/1471-2164-14-632
18. Swindell, WR, Johnston, A, Voorhees, JJ, Elder, JT, and Gudjonsson, JE. Dissecting the psoriasis transcriptome: inflammatory- and cytokine-driven gene expression in lesions from 163 patients. BMC Genomics (2013). 14:527. doi:10.1186/1471-2164-14-527
19. Izar, B, Tirosh, I, Stover, EH, Wakiro, I, Cuoco, MS, Alter, I, et al. A single-cell landscape of high-grade serous ovarian cancer. Nat Med (2020). 26:1271–9. doi:10.1038/s41591-020-0926-0
Keywords: teratoma, expression profile, single cell, ovarian tumor, transcriptome
Citation: Shin S, Choi YJ, Jung S-H, Chung Y-J and Lee SH (2021) Single Cell Gene Transcriptome Analysis of Ovarian Mature Teratomas. Pathol. Oncol. Res. 27:604228. doi: 10.3389/pore.2021.604228
Received: 09 September 2020; Accepted: 26 February 2021;
Published: 16 April 2021.
Edited by:
József Tímár, Semmelweis University, HungaryCopyright © 2021 Shin, Choi, Jung, Chung and Lee. This is an open-access article distributed under the terms of the Creative Commons Attribution License (CC BY). The use, distribution or reproduction in other forums is permitted, provided the original author(s) and the copyright owner(s) are credited and that the original publication in this journal is cited, in accordance with accepted academic practice. No use, distribution or reproduction is permitted which does not comply with these terms.
*Correspondence: Yeun-Jun Chung, eWVqdW5AY2F0aG9saWMuYWMua3I=; Sug Hyung Lee, c3VodWxlZUBjYXRob2xpYy5hYy5rcg==