- 1Hepatobiliary Surgery, Hunan Provincial People’s Hospital (The First Affiliate Hospital of Hunan Normal University), Changsha, China
- 2The Medical Department, 3D Medicines Inc., Shanghai, China
- 3Department of Hepatobiliary Surgery, First Affiliated Hospital of Guangxi Medical University, Nanning, China
Background: Biliary tract cancer is a highly lethal malignancy with poor clinical outcome. Accumulating evidence indicates targeted therapeutics may provide new hope for improving treatment response in BTC, hence better understanding the genomic profile is particularly important. Since tumor tissue may not be available for some patients, a complementary method is urgently needed. Circulating tumor DNA (ctDNA) provides a noninvasive means for detecting genomic alterations, and has been regarded as a promising tool to guide clinical therapies.
Methods: Next-generation sequencing of 150 cancer-related genes was used to detect gene alterations in blood-derived ctDNA from 154 Chinese patients with BTC. Genomic alterations were analyzed and compared with an internal tissue genomic database and TCGA database.
Results: 94.8% patients had at least one change detected in their ctDNA. The median maximum somatic allele frequency was 6.47% (ranging 0.1–34.8%). TP53 and KRAS were the most often mutated genes. The frequencies of single nucleotide variation in commonly mutated genes in ctDNA were similar to those detected in tissue samples, TP53 (35.1 vs. 40.4%) and KRAS (20.1 vs. 22.6%). Pathway analysis revealed that mutated genes were mapped to several key pathways including PI3K-Akt, p53, ErbB and Ras signaling pathway. In addition, patients harboring LRP1B, TP53, and ErbB family mutations presented significantly higher tumor mutation burden.
Conclusions: These findings demonstrated that ctDNA testing by NGS was feasible in revealing genomic changes and could be a viable alternative to tissue biopsy in patients with metastatic BTC.
Background
Biliary tract cancer (BTC) is a heterogeneous group of malignancies including intrahepatic cholangiocarcinoma (IHC), extrahepatic cholangiocarcinoma (EHC) and gallbladder cancer (GBC), which account for 3% of gastrointestinal malignancies [1,2]. Despite being rare in western countries, the incidence of BTC is increasing worldwide [3,4]. BTC is an aggressive disease with a dismal prognosis [5]. Complete surgical resection provides the only chance for cure, but only 10% of patients are diagnosed at early-stage disease and are suitable for resection [6]. In addition, the recurrence rate is relatively high [7,8]. Thus, for the majority of BTC patients, systemic chemotherapy is the mainstay of treatment. Gemcitabine plus cisplatin (GemCis) is the standard regimen for first-line treatment, but the objective response rate is about 20% and the survival gain is limited [9]. These highlight the need for the development of more effective treatment strategies.
Several molecular profiling studies have characterized the genomic landscape of BTC and indicated potentially targetable genomic alterations, including IDH1 mutations, FGFR2 fusions, BRAF mutations and so on [10,11]. Based on results of large clinical trials, targeted therapy drugs pemigatinib and ivosidenib have been approved by FDA to treat cholangiocarcinoma patients with FGFR2 fusions and IDH1 mutations, respectively [12,13]. These demonstrated the necessity that all patients underwent genetic testing prior to initiation of treatment.
While tissue biopsy remains the gold-standard, tissue may not be available or limited for some patients. And the inter and intratumor heterogeneity is another pivotal challenge [14,15]. Liquid biopsy has emerged as a strategy to these challenges by detecting circulating tumor DNA (ctDNA) [16]. It is becoming a widely used diagnostic tool for identifying genomic alterations to guide therapy and prognosis. In BTC, several researches have been launched on assessing the sensitivity and positive predict value of ctDNA. Kinugasa et al. [17] revealed that there was high concordance rate between bile ctDNA and tissue DNA samples and ctDNA might be used as a tool to diagnose gallbladder cancer. In addition, changes in cell-free DNA correlated well with tumor marker dynamics in pancreatobiliary carcinoma, thus demonstrating the feasibility of cfDNA sequencing in identifying tumor-derived mutations [22].
In this study, we identified genomic alterations in blood-derived ctDNA from patients with BTC and assessed the concordance between alterations from ctDNA and tumor tissue DNA. Our aim is to prove that blood-derived ctDNA sequencing could be a potential complement to tissue testing, and might guide personalized cancer treatment.
Materials and Methods
Sample Collection and Clinicopathologic Data
From January 2017 to December 2018, blood samples from 154 patients and tumor specimens from 545 patients with metastatic BTC were collected for tumor genomic DNA (gDNA) sequencing in Hunan Provincial People’s Hospital. The parallel blood samples of those 545 tumor tissues were also collected to identify normal genomic DNA sequences. Hunan Provincial People’s Hospital Medical ethics committee approved this study (2019 Scientific Research Ethics Review NO: 71), and all patients signed the waiver of informed consent form. All these samples were sent to a commercial company owning a CLIA-accredited/CAP-certified laboratory (3D Medicines Inc., Shanghai, China) for gene panel sequencing. In addition, the clinicopathologic characteristics, age and sex, were collected.
DNA Isolation and Sequencing
The methods of DNA extraction, sequencing and data analysis obeyed the published descriptions with some modifications [37]. Briefly, venous blood in STRECK tubes was centrifuged and kept the upper layer for the following tumor gDNA extraction via using the QIAamp Circulating Nucleic Acid Kit (Qiagen, Germany). The cfDNA libraries were established by Accel-NGS 2S Plus DNA Library Kit (Swift BioSciences, United States), and then sequenced. The gDNA of tissue sample with quality control and white blood cells were extracted by the DNeasy Tissue or Blood Kit (Qiagen, Germany), respectively. After fragmenting gDNA, the sequencing libraries were prepared by KAPA Hyper Prep Kit (KAPA Biosystems, United States). After capturing, the libraries were loaded into NextSeq500 platform (Illumina, United States) and performed next-generation sequencing (targeted) 150 cancer-related genes [18]. After eliminating duplicate or redundant information, the average coverage depth was 3000× for ctDNA and 500× for tissue sample.
Data and Statistical Analysis
Sequencing reads were mapped to the GRCh37/hg19 human reference genome, and analyzed for somatic genomic alterations (GAs) including single nucleotide variant (SNV), copy number variation (CNV) and fusion. The range of maximum somatic allele frequency (MSAF) was defined among 0.1 and 35% for all the somatic alterations per sample. Variants of unknown significance was included for calculating MASF, however nor was single nucleotide polymorphism. Clinically relevant GAs were defined as GAs that associated with response to currently available therapies or in target-driven clinical trials. TMB was defined as total number of somatic non-synonymous mutations in coding region. The raw data that support the findings of this study are available from the corresponding author upon reasonable request. In addition, data from the Cancer Genome Atlas (TCGA, https://www.cbioportal.org/) was extracted in December 2018 [19,20]. Gene Oncology (GO) and pathway analysis on gene alterations from ctDNA were performed using DAVID (https://david.ncifcrf.gov/) with the parameters p value cutoff = 0.05, and drawn in R by using the package “ggplot”.
Demographic characteristics of patients were analyzed using the T test or Chi-Square (χ2) test. Two sided p-values were evaluated and p < 0.05 was regarded as significance with statistical meaning. All the statistical analyses were performed by SPSS software, version 20.0 (SPSS Inc®, United States).
Results
Patient Characteristics and Basic Features of Genomic Alterations
Hybrid capture-based genomic profiling were performed on ctDNA samples and tumor tissue DNA samples, respectively (Table 1). For patients who provided ctDNA samples, the median age was 61 years, ranging from 39 to 93 years old. Among them 66.2% were male. Cholangiocarcinoma was the most common pathologic subtype (72.1%), followed by gallbladder cancer (24.0%), and others (3.9%) such as ampullary carcinoma. ctDNA in the blood was detected in 94.8% of the cases as approximated using a maximum somatic allele frequency (MSAF) > 0. The median MSAF was 6.47% (range 0.1–34.8%) and the average number of GAs was 4. As shown in Figure 1, highest median MSAF was observed in patients with gallbladder cancer, followed by patients with other pathological types and those with cholangiocarcinoma (p < 0.05) (Figure 1A). Male patients showed significantly higher median MSAF compared to female patients (p = 0.0001) (Figure 1B) while age had no significant effect (Figure 1C). For patients who provided tumor tissue samples, the median age was 59 years, ranging from 19 to 83 years old. The gender composition is relatively close to balance, with a distribution of 56.1% male and 43.9% female. Similar pathological types were observed, and cholangiocarcinoma (67.3%) was the dominant one. For patients providing paired tumor tissue and blood samples, ctDNA in the blood was detected in 520 (95.4%) of them and the median MSAF of tissue DNA was a little higher than that of ctDNA. The average GAs was 5. No significant correlation was observed between diverse baseline characteristics and TMB, including pathological subtype, sex and age (Figures 1D–F).
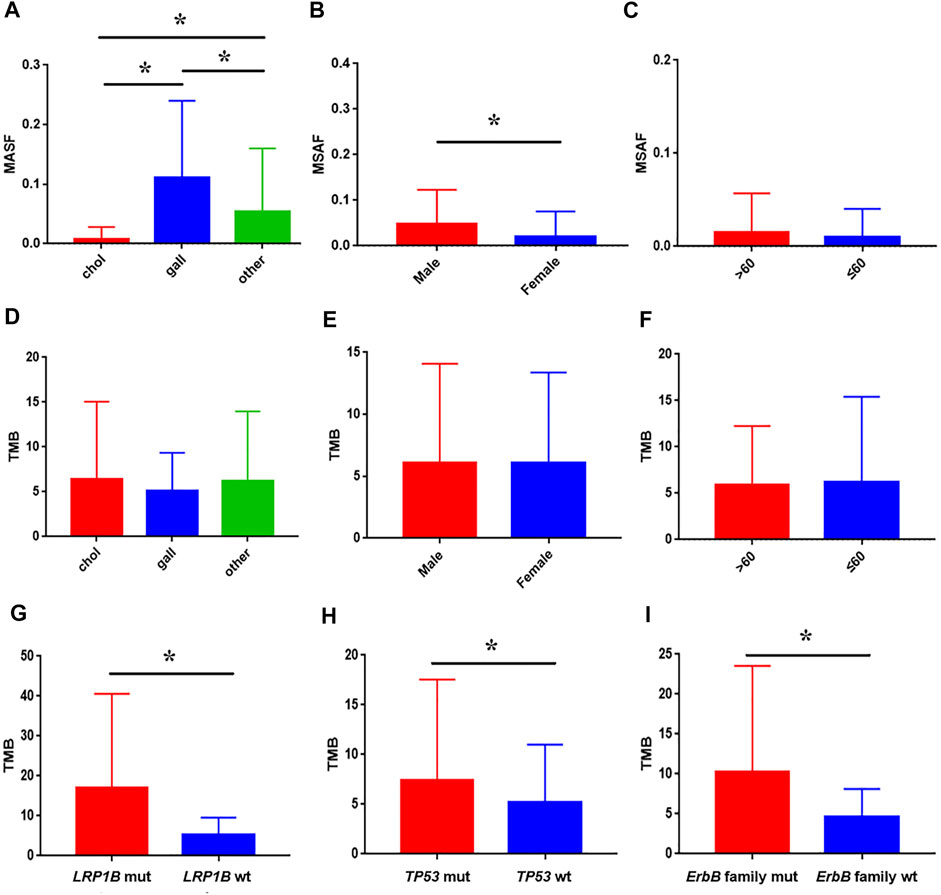
FIGURE 1. Association between baseline characteristics and ctDNA alteration in clinical samples. The impact of pathological subtype (A), sex (B), and age (C) on MSAF; the impact of pathological subtype (D), sex (E), and age (F) on TMB; comparison of TMB between patients with LRP1B (G), TP53 (H), ErbB family (I) mutation and wild-type, respectively.
Genomic Alterations in Blood-Derived ctDNA
Genomic alterations in ctDNA samples were identified using unique barcoding markers (Figure 2). TP53 (35.1%) and KRAS (20.1%) were found to be the most frequently altered genes. Using MutSigCV, other driver genes were also identified including EGFR (15.6%) and CDKN2A (9.7%). In 105 patients with cholangiocarcinoma, TP53 was the most frequently altered gene in ctDNA, followed by KRAS and EGFR (Figure 3A). By contrast, 37 blood samples with gallbladder subtypes were significantly enriched for TP53, CDKN2A, and EGFR mutations (Figure 3B). Patients harboring LRP1B, TP53, and ErbB family mutations showed significantly higher tumor mutation burden (TMB, Figures 1G–I).
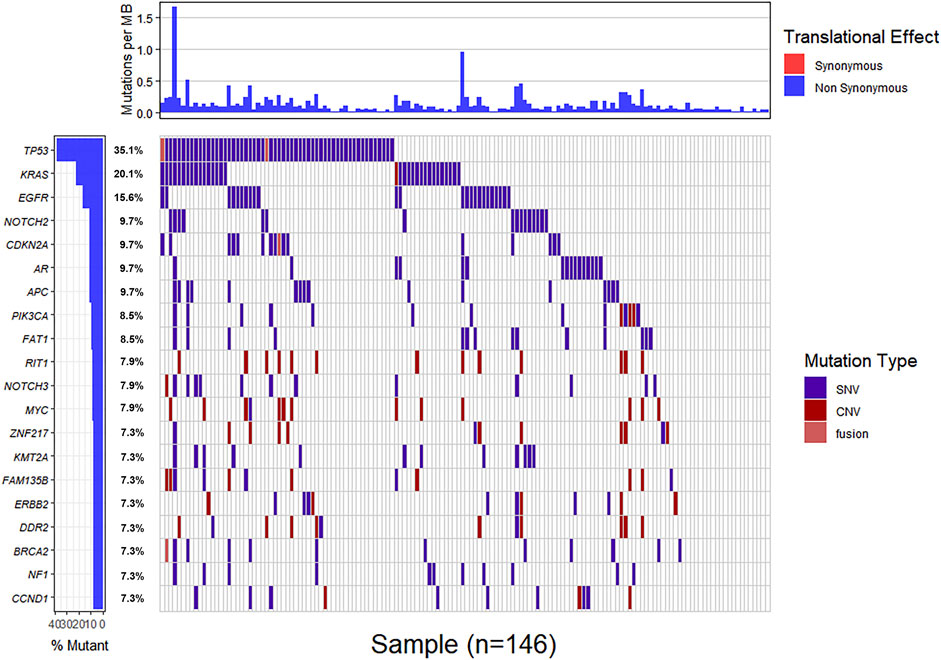
FIGURE 2. The mutation landscape of ctDNA samples. Mutations of genes in each sample were seen in the waterfall plot where various colors describing the specific forms of mutations were annotated.
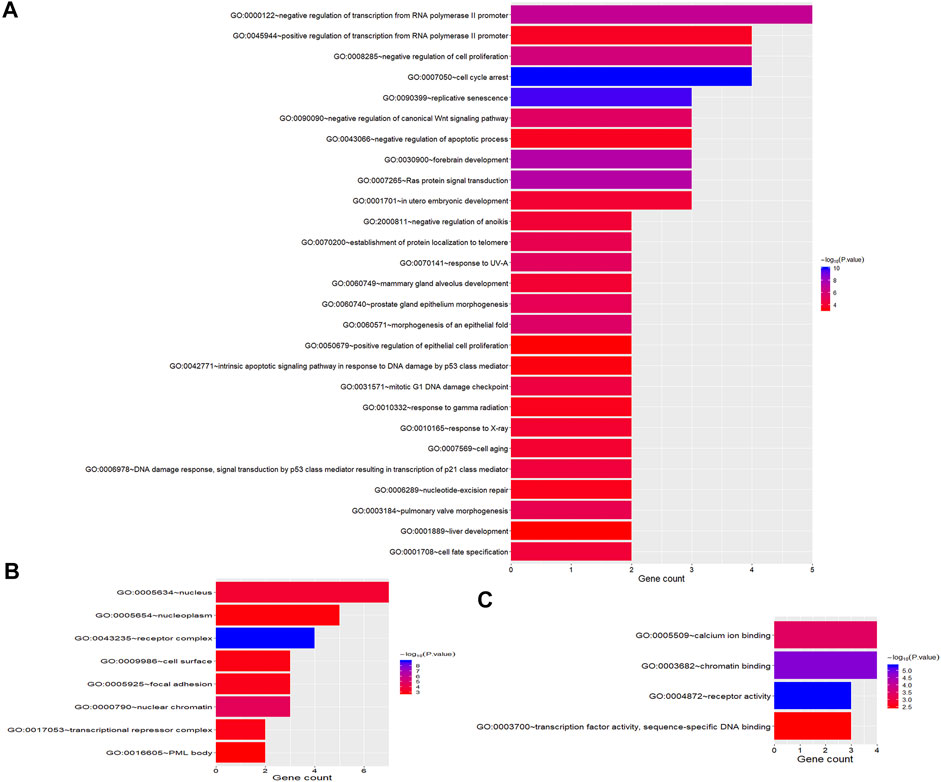
FIGURE 3. The mutation landscape of ctDNA in cholangiocarcinoma (A) and gallbladder (B) samples. Mutations of genes in each sample were seen in the waterfall plot where various colors describing the specific forms of mutations were annotated.
GO Enrichment and Signaling Pathway Analysis of Genomic Alterations
To better understand the biological function of these frequent alterations, gene ontology enrichment and signaling pathway analysis were performed. Figure 4 showed the significant enriched GO terms on three aspects, namely biological process (BP), cellular component (CC), and molecular function (MF). The top one enriched GO terms of BP was related to cell cycle and regulation, including regulation of transcription, regulation of cell proliferation, and cell cycle arrest. Most of the genes located in the nucleus, and the MF of calcium ion binding and chromatin binding enriched the most number of genes. As shown in Figure 5, a number of pathways that may be implicated in BTC were commonly mapped, including PI3K-Akt signaling pathway, p53 signaling pathway, ErbB signaling pathway, and Ras signaling pathway. These pathways has been recognized to be associated with tumorigenesis and reported to be frequently mutated in BTC [10,11].
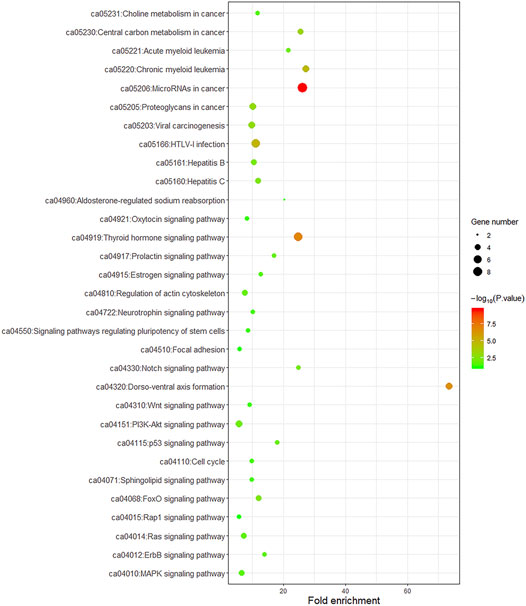
FIGURE 4. GO Enrichment Analysis of frequently mutated genes categorized by biological process (A), cellular component (B) and molecular function (C). The color represents the adjusted p-value.
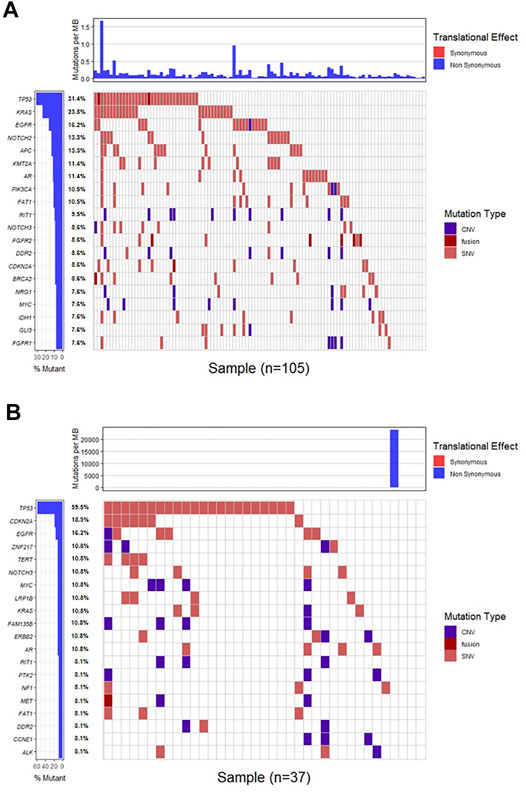
FIGURE 5. KEGG pathway enrichment dot plot of signaling pathways mapped by frequently mutated genes. The y-axis represents KEGG-enriched terms. The x-axis represents the fold of enrichment. The size of the dot represents the number of genes under a specific term. The color of the dots represents the adjusted p-value.
Comparison of Alterations in ctDNA Versus Tissue and TCGA Database
The frequencies of SNVs in commonly mutated genes in ctDNA samples were compared with the frequencies detected in tissue samples and TGCA database (Figure 6). TP53 was the most commonly mutated gene in all the three data source (35.1 vs. 40.4% vs. 24.2%), followed by KRAS (20.1 vs. 22.6% vs. 10.1%). And for most genes the mutation frequencies in ctDNA were similar with those detected in tissue samples and were relative higher than in TCGA database, with the exception of ARID1A and IDH1 which were most highly mutated in TCGA database.
Discussion
Somatic mutations were analyzed in blood samples of patients with advanced BTC, and ctDNA somatic mutations could be detected in 94.8% of all the cases. This result is consistent with other publications. Oliver et al. [21] reported the fraction is 84.6% (22/26) in patients with pancreatobiliary carcinomas. In our study, TP53 and KRAS were the most frequently mutated genes, followed by EGFR. A whole-exome and targeted gene sequencing result identified that genes with a significantly frequency of mutations included TP53 (47.1%) and KRAS (7.8%); the ErbB signaling was the most extensively mutated pathway affecting 36.8% of the GBC patients [20]. TP53 and KRAS were also identified as the significantly mutated genes in a cohort of ICC patients and Ras/PI3K signaling was one of the most affected pathways, followed by cell cycle signaling pathway [22]. These are basically consistent with our results on GO and pathway analysis.
IDH1, FGFR2 and BRAF are targetable genetic alterations in BTC. In our cohort, the frequencies of IDH1 mutations and FGFR2 fusions detected by ctDNA profiling in cholangiocarcinoma were 7.4 and 4.8%, respectively. These were very close to the mutational frequencies in tissue-based testing, which were 6 and 2.7%, respectively. We did not see any case harboring BRAF V600E mutation, a rare occurrence restricted in intrahepatic cholangiocarcinoma [23]. These indicate that ctDNA analysis, an alternative for tissue analysis, might be helpful to guide clinical decision in advanced BTC.
We identified that there was no significant difference on TMB among diverse pathological subtypes, which is consistent with the previous publication [24]. We also found that patients with LRP1B, TP53 or ErbB family member mutations had a significantly higher TMB than patients with wild-type genes respectively. LRP1B (low-density lipoprotein receptor-related protein 1B) gene mutations were frequently seen in multiple types of human cancer and had been recognized as driver mutations in liver cancer and pancreatic cancer [25–27]. Higher TMB was found in LRP1B mutated patients with melanoma and non-small cell lung cancer [28]. TP53 is a key tumor suppressor gene. The encoded protein plays a key role in the regulation of cell cycle arrest, apoptosis, senescence, DNA repair and changes in metabolism. Mutations in this gene are associated with a variety of human cancers [29]. An integrated analysis on the genomic, transcriptomic, proteomic, and clinical data from cohorts of lung adenocarcinoma patients revealed that TP53-mutated tumors showed prominently increased mutation burden [30]. Although several researches have discussed the relationship between LRP1B/TP53 gene mutation and TMB, no definite conclusions have been reached in BTC. The ErbB family of receptor tyrosine kinases comprises four members, ErbB-1/EGFR, ErbB-2/HER2, ErbB-3/HER3, ErbB-4/HER4. Mutation of these members occurred in nearly 15% of BTC patients and was associated with higher TMB [31]. This is consistent with our result.
The genomic landscape and molecular features of BTC have been reported in several papers [20,22,32]. However, almost all these researches use tumor tissues as the sequencing samples, and limited data on ctDNA profiling of BTC has been reported. On 2019 ASCO meeting, a research revealed the basic rudiment of the ctDNA genomic alteration landscape of BTC, and indicated that 55% of the patients harbored targetable genetic alterations [33]. Another blood-based genomic profiling also showed a subgroup of patients with BTC may benefit from targeted therapy and TP53 and KRAS were the most frequently altered genes [34]. In these papers the sequencing panel was relative small, thus may limit our understanding of genomic features. Researchers from Germany analyzed the correlation between ctDNA alterations and disease progression in BTC using a 710 cancer-related-genes panel [35]. However, only eight patients were detected by this panel, making the admissibility of the result quite weak.
To our knowledge, this work firstly revealed the genomic landscape of ctDNA in BTC with a large sample size, and directly compared it with the genomic landscape of tumor tissue DNA. These results indicated that ctDNA could be used as a potential complementary tool for gene sequencing, aiding to screen patients who may benefit from targeted therapies.
One limitation of this paper is the lack of baseline clinical characteristics and therapeutic regimens. As the clinical features may affect the detection of ctDNA [36,37], further studies are needed to study the association between clinical information and molecular information.
Data Availability Statement
The datasets presented in this article are not readily available as the ethical approval did not cover public disclosure of the data. Requests to access the datasets should be directed to HS,dG50Ym95MTk4MkAxNjMuY29t.
Ethics Statement
The studies involving human participants were reviewed and approved by Hunan Provincial People’s Hospital Medical ethics committee. The patients/participants provided their written informed consent to participate in this study.
Author Contributions
HS and CC designed the study. JS and MY wrote the manuscript. TW, MY, JS, MH, and YB were involved in data collection, statistical analyses and interpretation of data. All authors read and approved the final manuscript.
Funding
The work was supported by grants from the Hunan province technical innovation guidance program-clinical medical technology innovation guidance project (2018SK50708), Scientific research plan project of Education Bureau of Hunan Province (19C1183) and Scientific research plan project of Health Committee of Hunan Province (C2016005).
Abbreviations
BP, Biological process; BTC, Biliary tract cancer; CC, cfDNA Cellular component Cell-free DNA; CNV, Copy number variation; ctDNA, Circulating tumor DNA; GA, Genomic alteration; gDNA, Genomic DNA; GO, Gene oncology; ICI, Immune checkpoint inhibitor; LRP1B, Low-density lipoprotein receptor-related protein 1B; MF, Molecular function; MSAF, Maximum somatic allele frequency; SNV, Single nucleotide variant; TCGA, The Cancer Genome Atlas; TMB, Tumor mutation burden.
Conflict of Interest
Authors MY, JS, MH, and YB were employed by the company 3D Medicines Inc.
The remaining authors declare that the research was conducted in the absence of any commercial or financial relationships that could be construed as a potential conflict of interest.
References
1. Goodman, MT, and Yamamoto, J. Descriptive Study of Gallbladder, Extrahepatic Bile Duct, and Ampullary Cancers in the United States, 1997-2002. Cancer Causes Control (2007) 18(4):415–22. doi:10.1007/s10552-006-0109-4
2. Fornaro, L, Cereda, S, Aprile, G, Di Girolamo, S, Santini, D, Silvestris, N, et al. Multivariate Prognostic Factors Analysis for Second-Line Chemotherapy in Advanced Biliary Tract Cancer. Br J Cancer (2014) 110(9):2165–9. doi:10.1038/bjc.2014.190
3. Jepsen, P, Vilstrup, H, Tarone, RE, Friis, S, and Sorensen, HT. Incidence Rates of Intra- and Extrahepatic Cholangiocarcinomas in Denmark from 1978 through 2002. JNCI J Natl Cancer Inst (2007) 99(11):895–7. doi:10.1093/jnci/djk201
4. Shaib, YH, Davila, JA, McGlynn, K, and El-Serag, HB. Rising Incidence of Intrahepatic Cholangiocarcinoma in the United States: a True Increase. J Hepatol (2004) 40(3):472–7. doi:10.1016/j.jhep.2003.11.030
5. Athauda, A, Fong, C, Lau, DK, Javle, M, Abou-Alfa, GK, Morizane, C, et al. Broadening the Therapeutic Horizon of Advanced Biliary Tract Cancer through Molecular Characterisation. Cancer Treat Rev (2020) 86:101998. doi:10.1016/j.ctrv.2020.101998
6. Hezel, AF, and Zhu, AX. Systemic Therapy for Biliary Tract Cancers. Oncol (2008) 13(4):415–23. doi:10.1634/theoncologist.2007-0252
7. Wang, SJ, Lemieux, A, Kalpathy-Cramer, J, Ord, CB, Walker, GV, Fuller, CD, et al. Nomogram for Predicting the Benefit of Adjuvant Chemoradiotherapy for Resected Gallbladder Cancer. Jco (2011) 29(35):4627–32. doi:10.1200/jco.2010.33.8020
8. Wang, Y, Li, J, Xia, Y, Gong, R, Wang, K, Yan, Z, et al. Prognostic Nomogram for Intrahepatic Cholangiocarcinoma after Partial Hepatectomy. Jco (2013) 31(9):1188–95. doi:10.1200/jco.2012.41.5984
9. Malka, D, Cervera, P, Foulon, S, Trarbach, T, de la Fouchardière, C, Boucher, E, et al. Gemcitabine and Oxaliplatin with or without Cetuximab in Advanced Biliary-Tract Cancer (BINGO): a Randomised, Open-Label, Non-comparative Phase 2 Trial. Lancet Oncol (2014) 15(8):819–28. doi:10.1016/s1470-2045(14)70212-8
10. Nakamura, H, Arai, Y, Totoki, Y, Shirota, T, Elzawahry, A, Kato, M, et al. Genomic Spectra of Biliary Tract Cancer. Nat Genet (2015) 47(9):1003–10. doi:10.1038/ng.3375
11. Jusakul, A, Cutcutache, I, Yong, CH, Lim, JQ, Huang, MN, Padmanabhan, N, et al. Whole-Genome and Epigenomic Landscapes of Etiologically Distinct Subtypes of Cholangiocarcinoma. Cancer Discov (2017) 7(10):1116–35. doi:10.1158/2159-8290.cd-17-0368
12. Abou-Alfa, GK, Macarulla, T, Javle, MM, Kelley, RK, Lubner, SJ, Adeva, J, et al. Ivosidenib in IDH1-Mutant, Chemotherapy-Refractory Cholangiocarcinoma (ClarIDHy): a Multicentre, Randomised, Double-Blind, Placebo-Controlled, Phase 3 Study. Lancet Oncol (2020) 21(6):796–807. doi:10.1016/s1470-2045(20)30157-1
13. Abou-Alfa, GK, Sahai, V, Hollebecque, A, Vaccaro, G, Melisi, D, Al-Rajabi, R, et al. Pemigatinib for Previously Treated, Locally Advanced or Metastatic Cholangiocarcinoma: a Multicentre, Open-Label, Phase 2 Study. Lancet Oncol (2020) 21(5):671–84. doi:10.1016/s1470-2045(20)30109-1
14. Hiley, C, de Bruin, EC, McGranahan, N, and Swanton, C. Deciphering Intratumor Heterogeneity and Temporal Acquisition of Driver Events to Refine Precision Medicine. Genome Biol (2014) 15(8):453. doi:10.1186/s13059-014-0453-8
15. Gerlinger, M, Rowan, AJ, Horswell, S, Math, M, Larkin, J, Endesfelder, D, et al. Intratumor Heterogeneity and Branched Evolution Revealed by Multiregion Sequencing. N Engl J Med (2012) 366(10):883–92. doi:10.1056/NEJMoa1113205
16. Chae, YK, Davis, AA, Jain, S, Santa-Maria, C, Flaum, L, Beaubier, N, et al. Concordance of Genomic Alterations by Next-Generation Sequencing in Tumor Tissue versus Circulating Tumor DNA in Breast Cancer. Mol Cancer Ther (2017) 16(7):1412–20. doi:10.1158/1535-7163.mct-17-0061
17. Kinugasa, H, Nouso, K, Ako, S, Dohi, C, Matsushita, H, Matsumoto, K, et al. Liquid Biopsy of Bile for the Molecular Diagnosis of Gallbladder Cancer. Cancer Biol Ther (2018) 19(10):934–8. doi:10.1080/15384047.2018.1456604
18. Wang, Z, Duan, J, Cai, S, Han, M, Dong, H, Zhao, J, et al. .Assessment of Blood Tumor Mutational Burden as a Potential Biomarker for Immunotherapy in Patients with Non-small Cell Lung Cancer with Use of a Next-Generation Sequencing Cancer Gene Panel. JAMA Oncol (2019) 5(5):696–702. doi:10.1001/jamaoncol.2018.7098
19. Lowery, MA, Ptashkin, R, Jordan, E, Berger, MF, Zehir, A, Capanu, M, et al. Comprehensive Molecular Profiling of Intrahepatic and Extrahepatic Cholangiocarcinomas: Potential Targets for Intervention. Clin Cancer Res (2018) 24(17):4154–61. doi:10.1158/1078-0432.ccr-18-0078
20. Li, M, Zhang, Z, Li, X, Ye, J, Wu, X, Tan, Z, et al. Whole-exome and Targeted Gene Sequencing of Gallbladder Carcinoma Identifies Recurrent Mutations in the ErbB Pathway. Nat Genet (2014) 46(8):872–6. doi:10.1038/ng.3030
21. Zill, OA, Greene, C, Sebisanovic, D, Siew, LM, Leng, J, Vu, M, et al. Cell-Free DNA Next-Generation Sequencing in Pancreatobiliary Carcinomas. Cancer Discov (2015) 5(10):1040–8. doi:10.1158/2159-8290.cd-15-0274
22. Zou, S, Li, J, Zhou, H, Frech, C, Jiang, X, Chu, JSC, et al. Mutational Landscape of Intrahepatic Cholangiocarcinoma. Nat Commun (2014) 5:5696. doi:10.1038/ncomms6696
23. Goeppert, B, Frauenschuh, L, Renner, M, Roessler, S, Stenzinger, A, Klauschen, F, et al. BRAF V600E-specific Immunohistochemistry Reveals Low Mutation Rates in Biliary Tract Cancer and Restriction to Intrahepatic Cholangiocarcinoma. Mod Pathol (2014) 27(7):1028–34. doi:10.1038/modpathol.2013.206
24. Abdel-Wahab, R, and Mahamed-Ali, S. Variations in DNA Repair Genomic Alterations and Tumor Mutation burden in Biliary Tract Cancer (BTC) Subtypes. ASCO (2018) 36:263. doi:10.1200/jco.2018.36.4_suppl.263
25. Cotterchio, M, Lowcock, E, Bider-Canfield, Z, Lemire, M, Greenwood, C, Gallinger, S, et al. Association between Variants in Atopy-Related Immunologic Candidate Genes and Pancreatic Cancer Risk. PLoS One (2015) 10(5):e0125273. doi:10.1371/journal.pone.0125273
26. Ding, D, Lou, X, Hua, D, Yu, W, Li, L, Wang, J, et al. Recurrent Targeted Genes of Hepatitis B Virus in the Liver Cancer Genomes Identified by a Next-Generation Sequencing-Based Approach. Plos Genet (2012) 8(12):e1003065. doi:10.1371/journal.pgen.1003065
27. Liu, C-X, Li, Y, Obermoeller-McCormick, LM, Schwartz, AL, and Bu, G. The Putative Tumor Suppressor LRP1B, a Novel Member of the Low Density Lipoprotein (LDL) Receptor Family, Exhibits Both Overlapping and Distinct Properties with the LDL Receptor-Related Protein. J Biol Chem (2001) 276(31):28889–96. doi:10.1074/jbc.m102727200
28. Chen, H, Chong, W, Wu, Q, Yao, Y, Mao, M, and Wang, X. Association of LRP1B Mutation with Tumor Mutation Burden and Outcomes in Melanoma and Non-small Cell Lung Cancer Patients Treated with Immune Check-Point Blockades. Front Immunol (2019) 10:1113. doi:10.3389/fimmu.2019.01113
29. Kruiswijk, F, Labuschagne, CF, and Vousden, KH. p53 in Survival, Death and Metabolic Health: a Lifeguard with a Licence to Kill. Nat Rev Mol Cel Biol (2015) 16(7):393–405. doi:10.1038/nrm4007
30. Dong, Z-Y, Zhong, W-Z, Zhang, X-C, Su, J, Xie, Z, Liu, S-Y, et al. Potential Predictive Value of TP53 and KRAS Mutation Status for Response to PD-1 Blockade Immunotherapy in Lung Adenocarcinoma. Clin Cancer Res (2017) 23(12):3012–24. doi:10.1158/1078-0432.ccr-16-2554
31. Zhang, Y, Shen, N, Yu, W, and Fu, X. Tumor Mutation burden in Biliary Tract Cancers with ERBB Family or DNA Damage Repair Gene Mutations. ASCO (2019) 37(15):e15602. doi:10.1200/JCO.2019.37.15_suppl.e15602
32. Valle, JW, Lamarca, A, Goyal, L, Barriuso, J, and Zhu, AX. New Horizons for Precision Medicine in Biliary Tract Cancers. Cancer Discov (2017) 7(9):943–62. doi:10.1158/2159-8290.cd-17-0245
33. Mody, K, Kasi, PM, Yang, J, Surapaneni, PK, Bekaii-Saab, T, Ahn, DH, et al. Circulating Tumor DNA Profiling of Advanced Biliary Tract Cancers. JCO Precision Oncol (2019) 3:1–9. doi:10.1200/po.18.00324
34. Goyal, L, Kelley, RK, Kiedrowski, L, Catenacci, D, Mody, K, Shroff, R, and Parikh, A. Blood-based Genomic Profiling of Cell-free Tumor DNA (ctDNA) in Patients with Biliary Tract Cancer. In: Proceedings of the AACR-NCI-EORTC International Conference; October 26-30, 2017; Philadelphia, PA. Philadelphia, PA: Molecular Targets and Cancer Therapeutics (2017). doi:10.1158/1535-7163
35. Ettrich, TJ, Schwerdel, D, Dolnik, A, Beuter, F, Blätte, TJ, Schmidt, SA, et al. Genotyping of Circulating Tumor DNA in Cholangiocarcinoma Reveals Diagnostic and Prognostic Information. Sci Rep (2019) 9(1):13261. doi:10.1038/s41598-019-49860-0
36. Yang, N, Li, Y, Liu, Z, Qin, H, Du, D, Cao, X, et al. The Characteristics of ctDNA Reveal the High Complexity in Matching the Corresponding Tumor Tissues. BMC Cancer (2018) 18(1):319. doi:10.1186/s12885-018-4199-7
Keywords: next-generation sequencing, signaling pathway, circulating tumor DNA, biliary tract cancer, genomic feature
Citation: Chen C, Wang T, Yang M, Song J, Huang M, Bai Y and Su H (2021) Genomic Profiling of Blood-Derived Circulating Tumor DNA from Patients with Advanced Biliary Tract Cancer. Pathol. Oncol. Res. 27:1609879. doi: 10.3389/pore.2021.1609879
Received: 13 May 2021; Accepted: 30 September 2021;
Published: 15 October 2021.
Edited by:
József Tímár, Semmelweis University, HungaryCopyright © 2021 Chen, Wang, Yang, Song, Huang, Bai and Su. This is an open-access article distributed under the terms of the Creative Commons Attribution License (CC BY). The use, distribution or reproduction in other forums is permitted, provided the original author(s) and the copyright owner(s) are credited and that the original publication in this journal is cited, in accordance with accepted academic practice. No use, distribution or reproduction is permitted which does not comply with these terms.
*Correspondence: Hao Su, dG50Ym95MTk4MkAxNjMuY29t
†These authors share first authorship